
Guided by senior executive goals for digital transformation, more organizations are increasing their use of cloud computing technologies. The typical multi-cloud mix includes a blend of public cloud, private cloud and traditional IT services. Finding the best-fit service mix starts with the business requirements.
The latest Global Cloud Index (GCI) 2016-2021 from Cisco focuses on the worldwide market outlook for enterprise data centre virtualization and cloud computing services. Today's digital business is enabled by Hybrid IT infrastructure that supports the deployment of cloud-based solutions.
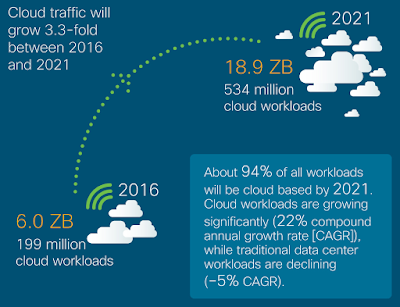
Driven by the surging enthusiasm for digital reinvention projects, data centre traffic is growing fast. The market study authors forecast global cloud data centre traffic will reach 19.5 zettabytes (ZB) per year by 2021 — that's up from 6.0 ZB per year in 2016.
Globally, cloud data centre traffic will represent 95 percent of total data centre traffic by 2021, compared to 88 percent in 2016.
Additionally, according to the Cisco assessment, the growth of Internet of Things (IoT) applications requires scalable server and storage solutions to accommodate new and expanding data centre demands.
By 2021, Cisco expects IoT connections to reach 13.7 billion — that's up from 5.8 billion in 2016.
Hyperscale cloud data centre growth
By 2021 there will be 628 hyperscale data centres globally, compared to 338 in 2016 — that's 1.9-fold growth or near doubling over the forecast period. By 2021, hyperscale data centres will support:
- 53 percent of all data centre servers (27 percent in 2016)
- 69 percent of all data centre processing power (41 percent in 2016)
- 65 percent of all data stored in data centres (51 percent in 2016)
- 55 percent of all data centre traffic (39 percent in 2016)
Data centre virtualization and cloud growth
By 2021, 94 percent of workloads and compute instances will be processed by cloud data centres. In contrast, 6 percent will be processed by traditional IT data centres.
Overall data centre workloads and compute instances will more than double (2.3-fold) from 2016 to 2021; however, cloud workloads and compute instances will nearly triple (2.7-fold) over the same period.
The workload and compute instance density for cloud data centres was 8.8 in 2016 and will grow to 13.2 by 2021. Comparatively, for traditional data centres, workload and compute instance density was 2.4 in 2016 and will grow to 3.8 by 2021.
Stored data growth fuelled by big data and IoT
Globally, the data stored in data centres will nearly quintuple by 2021 to reach 1.3 ZB by 2021, up 4.6-fold (a CAGR of 36 percent) from 286 EB in 2016.
Big data will reach 403 exabytes (EB) by 2021, up almost 8-fold from 25 EB in 2016. Big data will represent 30 percent of data stored in data centres by 2021, up from 18 percent in 2016.
The amount of data stored on devices will be 4.5 times higher than data stored in data centres, at 5.9 ZB by 2021.
Driven largely by IoT, the total amount of data created (and not necessarily stored) by any device will reach 847 ZB per year by 2021, up from 218 ZB per year in 2016. Data created is two orders of magnitude higher than data stored.
Apps contribute to rise of global data centre traffic
– By 2021, big data will account for 20 percent (2.5 ZB annual, 209 EB monthly) of traffic within data centres, compared to 12 percent (593 EB annual, 49 EB monthly) in 2016.
– By 2021, video streaming will account for 10 percent of traffic within data centres, compared to 9 percent in 2016.
– By 2021, video will account for 85 percent of traffic from data centres to end users, compared to 78 percent in 2016.
– By 2021, search will account for 20 percent of traffic within data centres by 2021, compared to 28 percent in 2016.
– By 2021, social networking will account for 22 percent of traffic within data centres, compared to 20 percent in 2016
SaaS is still the most popular cloud service model
By 2021, 75 percent (402 million) of the total cloud workloads and compute instances will be SaaS workloads and compute instances, up from 71 percent (141 million) in 2016. (23 percent CAGR from 2016 to 2021).
By 2021, 16 percent (85 million) of the total cloud workloads and compute instances will be IaaS workloads and compute instances, down from 21 percent (42 million) in 2016. (15 percent CAGR from 2016 to 2021).
By 2021, 9 percent (46 million) of the total cloud workloads and compute instances will be PaaS workloads and compute instances, up from 8 percent (16 million) in 2016. (23 percent CAGR from 2016 to 2021).